Do you have a theory about something which may have a positive impact on your marketing? It might be time to run a test.
Great ideas can come to us at any time, when you’re reviewing marketing data, when you’re waiting at a red light, when your significant other is talking to you about something you’re not remotely interested in! Inspiration has struck, but how do you know if it’s a great idea or a non-starter? Time to experiment.
When it comes to marketing experiments, we’re generally talking about two types, A/B tests and multivariate testing (MVT). Before we delve into how to properly run a marketing experiment, let’s look at the differences between the two types.
What Is An A/B Test?
A/B testing, also known as split testing is the practice of splitting an audience straight down the middle, into Set A and Set B – hence the name. If we’re using this type of test for a landing page, Set A would see one version of the landing page. Set B would see the same landing page, but with one alteration made to it.
This type of testing is generally used when there is already existing live content, Set A would see the current content and Set B would see the altered version. That being said, there’s no harm is running a A/B tests when trying out a completely new email template for example, Set A would see a new email template and Set B would see the new email template with one alteration. All this means is that the data we receive will be difficult to decipher as there is no historic data to compare it against, what we would know is the difference between Set A and Set B on that specific occasion.
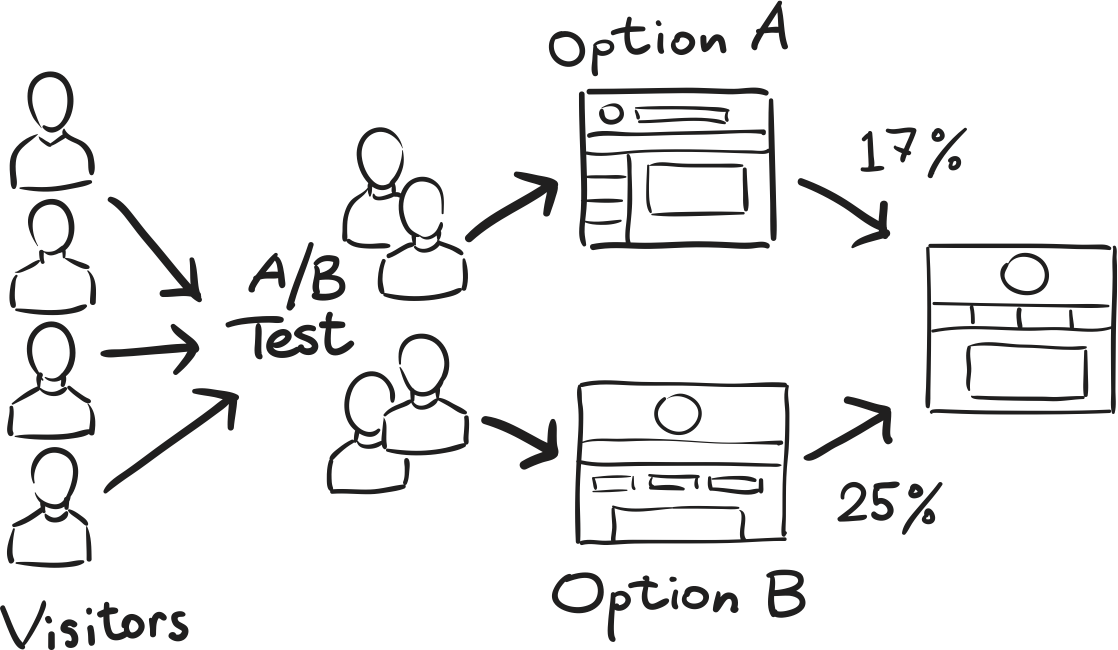
What is Multivariate Testing?
MVT testing is set up in a similar way to A/B tests, we have Version A (generally existing content) and Version B. The difference this time is that version B will have a whole array of alterations made to it. The versions are unlikely to look similar at all. MVT testing is best used when drastic alterations to content are made.
Often employed during a site refresh or a rebrand, MVT testing forms the first part helping to define how best to set up a landing page for example. A/B tests are then used to really fine tune the content. Combining MVT with A/B tests is common place when undergoing an exercise in conversion rate optimisation (CRO).
How To Set Up MVT & A/B Tests
Think back to when you were in school doing a science experiment, each experiment started with a hypothesis. These tests are no different begin with a strong hypothesis to test. For an A/B test, it may be that changing the position of a call-to-action (CTA) will increase conversion rates. For an MVT, the hypothesis is likely to be a little more in depth due to the nature of the testing process; nonetheless, it’s important to always begin with a hypothesis.
Once we have our theory in place, we can then enact them, for A/B tests this will be changing a specific element, for MVT there will be multiple, more radical changes.
It’s now time to define the audience, it could be that for certain tests, we’re only interested in a certain segments of the audience, for example hypothesis could be, “increasing the size of the CTA button by 30% will increase conversions in customers aged 45+”. Whatever your audience is, make sure it’s sizeable enough so there is actionable data – remember we are splitting the audience into two sets.
The next step is important, define your goals. More accurately, understand which metric you will be measuring – this isn’t always as cut and dry as conversion rates.
Now all that’s left to do is run the tests. If you’re running a test on Google ads, they have an experiment function. Likewise, MailChimp have an easy to use A/B testing functionality. In the event you cannot use a pre-formatted testing functionality, the test will need to run until you have enough statistically valid data.
Next Steps
It’s important to remember that A/B tests and MVT is an experiment – you might have a theory one way or another but you must trust the data. If results are inconclusive, it’s probably a good idea to retain the staus quo, changing things for the sake of changing them is more often than not an exercise in futility – put your time into something which will have a positive result.
There’s often a feeling that we have to do something after we’ve run a test, the reality though is that it not always needed. Are you unconvinced by the test results? Alter and test again. However, avoid repeat testing until you get the results which you think you should be getting – biased results defeat the whole object of A/B tests and MVT.
Action your results and continue to monitor. This last aspect is important, A/B tests and their MVT counterparts are great for proving or disproving a theory over a set period of time. However, what is the knock-on effect down the line? Perhaps there’s been a significant drop off in return custom? It’s vital to have your finger on the pulse of your data and constantly test & measure in order to stay ahead of the curve and keep them figures green.